Cancer Evolutionary Adaptation and Immune Evasion
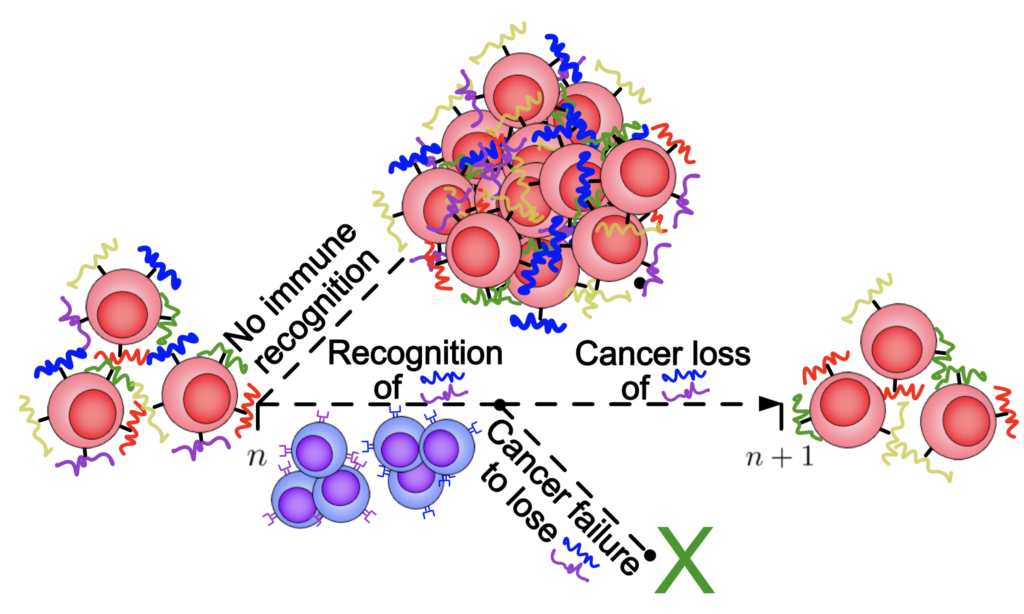
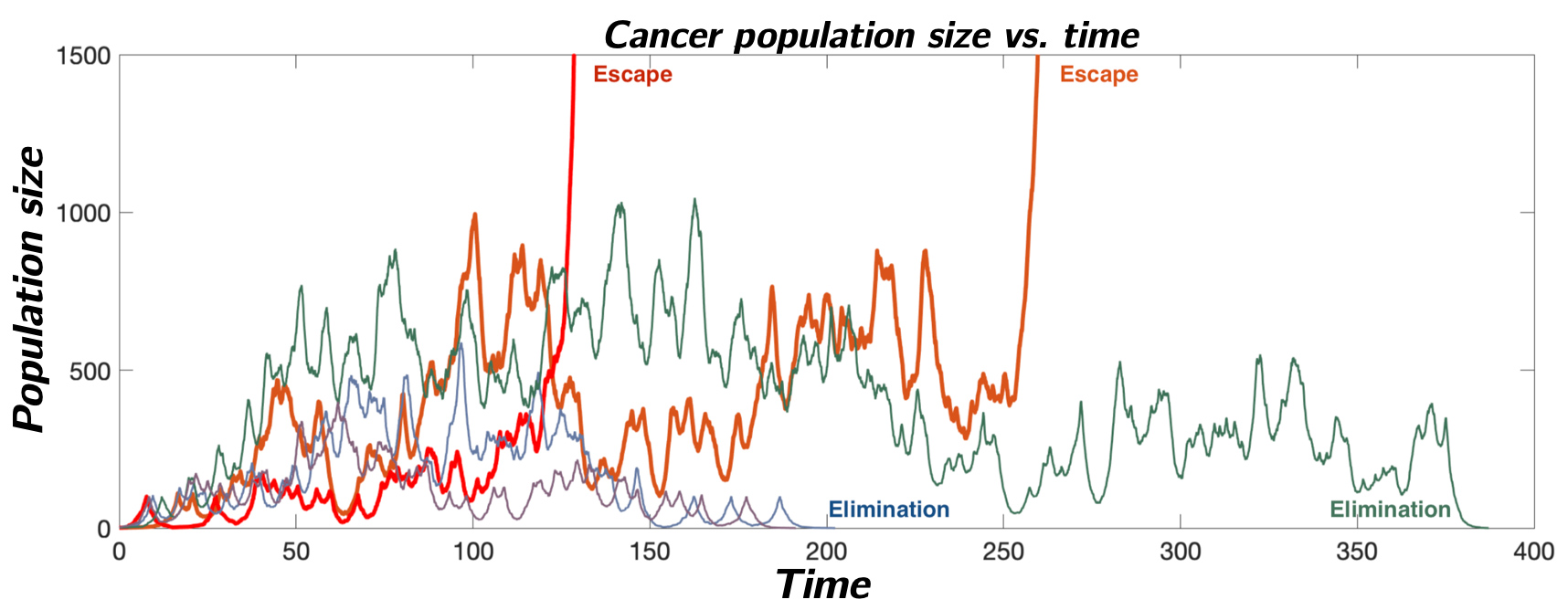
Cancer cells exhibit an elaborate array of mechanisms enabling them to effectively adapt to therapeutic intervention and acquire treatment resistance. The adaptive immune system provides a unique therapeutic opportunity to target cancer clones using millions of distinct T-cell receptors. Our group has been interested in developing theoretical models of the co-evolution between an evasive cancer population and a diverse repertoire of immune cells. Here, we employ stochastic birth-death process population dynamical models to link adaptive immune system recognition and cancer evolution to observed cancer evasion rates, and to identify drivers of cancer escape.
Repertoire-level Modeling of T cell Recognition
The human T cell repertoire consists of hundreds of millions of unique T cell clones, which gives it the extraordinary ability to recognize and eliminate new threats via the detection of antigens in the form of 9 to 11 amino acid peptide fragments presented on the surface of cells. During development, T cells are trained to avoid recognition of self-peptide. The impact of this tolerance on T cell recognition of non-self antigens – in particular, tumor neoantigens that are often point-mutated self-peptides – is critically important for optimizing cancer immunotherapy and predicting future immunogenic responses to viral variants. On the theoretical modeling front, we develop random energy frameworks for understanding how T cell tolerance affects self vs. non-self discrimination and the recognition of viral and tumor-associated antigens. Biophysical modeling of these interactions has led to the development of coarse-grained structural models capable of learning the interactions between antigens and T cell receptors that give rise to observed T cell specificity. These models are currently being applied to identify T cells specific for therapeutically relevant antigens in clinical collaboration.
SELECTED REFERENCES:
Wang, Lin, Ng Chau, Onuchic, Levine, George. Science Advances 2024.
Ng Chau, George, Lin, Onuchic, Levine. Physical Review E 2022.
Lin, George, Schafer, Ng Chau, Birnbaum, Clementi, Onuchic, Levine. Nature Computational Science 2021.
George, Kessler, Levine. Proceedings of the National Academy of Sciences USA 2017.
Inferential Modeling of the Epithelial to Mesenchymal Transition
Our research group develops and applies inferential modeling frameworks for characterizing phenotypic diversity, including cell populations undergoing an Epithelial to Mesenchymal Transition (EMT). EMT is a dynamic and reversible process that occurs during embryogenesis, wound healing, and also cancer progression. Our group pioneered one of the commonly used gene expression-based EMT scoring metrics to quantifying a biological sample’s location on the Epithelial-Mesenchymal (EM) phenotypic spectrum.
We have more recently identified a dynamical model of EMT progression using time course data to infer the forward and revere transition rates through EMT as a function of cell type and EMT induction. We continue to apply and refine these models through joint basic science and clinical collaboration, and are developing these models into open-source modeling tools for the scientific community.
SELECTED REFERENCES:
Najafi, Jolly, George. iScience 2023.
Mandal, Tejaswi, Janivara, Srikrishnan, Thakur, Sahoo, Chakraborty, Singh Sohal, Levine, George, Jolly. Biomolecules 2022.
Chakraborty, George, Tripathi, Levine, Jolly. Frontiers in Bioengineering and Biotechnology 2021.
George, Jolly, Xu, Somarelli, Levine. Cancer Research 2017.
Quantifying Phenotypic Adaptability in Fluctuating Environments
Phenotypic adaptation is a universal feature of biological systems navigating highly variable environments. The extent to which observed adaptation matches the optimal policy for cell populations faced with stochastic landscapes is unknown. Here, we develop foundational mathematical models to quantify adaptability and predict system response to temporally varying interventions. In the context of overcoming drug resistance, we are interested in applying these models to design improved treatment policies.